London Reporting House (LRH) has access to Securities Financing Transactions Regulation (SFTR) data that is otherwise only available to regulators. This allows its team to build a clearer picture of activity in the European repo market than individual participants.
One particular point of interest is the division of the repo market into cash-driven and collateral-driven transactions, that is, between so-called “general collateral” or GC repo and “specials.” Since GC repo is cash-driven, it should trade at or around a fixed repo rate – the GC repo rate. Special repo is securities-driven and trades at a wider range of different rates which depend on the intrinsic value of the collateral security. This intrinsic value drives special repo rates below the GC repo rate.
To determine which repos are GC and identify those that are trading special, LRH researchers have applied simple machine learning (ML) methods to an anonymized set of repo rates from a sample of actual transactions reported under SFTR.
They have characterized and compared the performance of these methods to one another, as well as to a simple, percentage-based cut-off method that is used in the market. All the ML methods implemented – Jenks Natural Breaks, Head/Tails, and Gaussian Mixture Models (GMM) – can classify trades in a manner that is robust to varying proportions of GC and specials in different market conditions. Head/Tails consistently outperforms current benchmarking methods, adapting to daily market conditions.
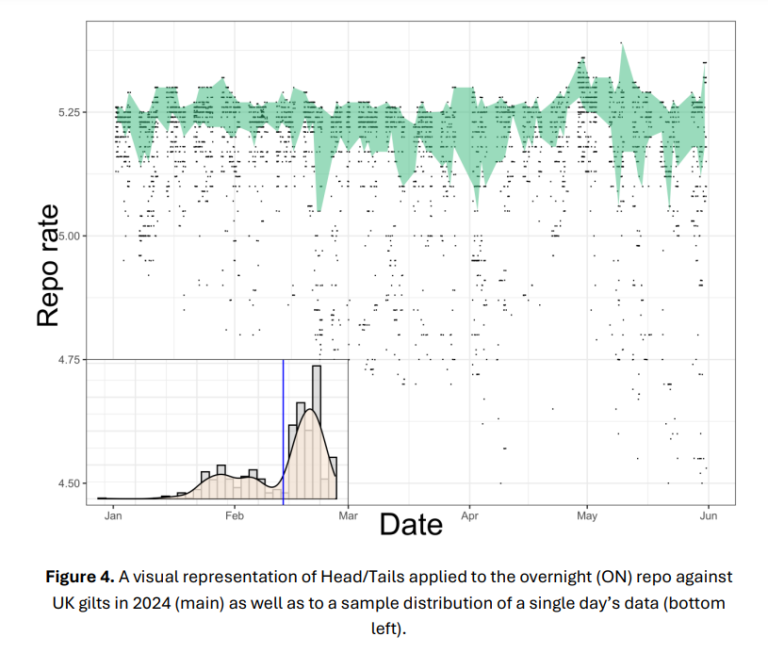
The novelty of rich, large datasets for the traditionally opaque repo market, such as the transaction-level SFTR data aggregated by LRH, provides an opportunity to reassess some of the existing methods that are used to measure the markets and calculate benchmarks. Even simple ML methods, such as those presented here, are able to leverage these sources to generate results that improve upon current industry standards.
The Head/Tails method is able to consistently split a distribution of repo rates into a GC ‘Head’ and a special ‘Tail’ in a manner that is robust to variation in the distribution of rates within the market, as well as the differences between markets and tenors. Researchers also present the Jenks and GMM models, that also outperform a simple threshold-based classification, though are less consistent for the single input variable than Head/Tails. These methods both represent a starting point for building more complex and sophisticated models.