A research note published by the Chartered Alternative Investment Analyst Association discusses how the adaptivity and self-learning capability of machine learning tools could add value along the entire value chain of an asset manager. The research note elaborates on two specific ways: first, by more effectively using currently available data based on algorithms that learn to reveal new, nonlinear relationships or by transforming data into representations with more interpretable meanings; and second, by embracing new data sources that provide additional information, for example, based on news flow and blog posts.
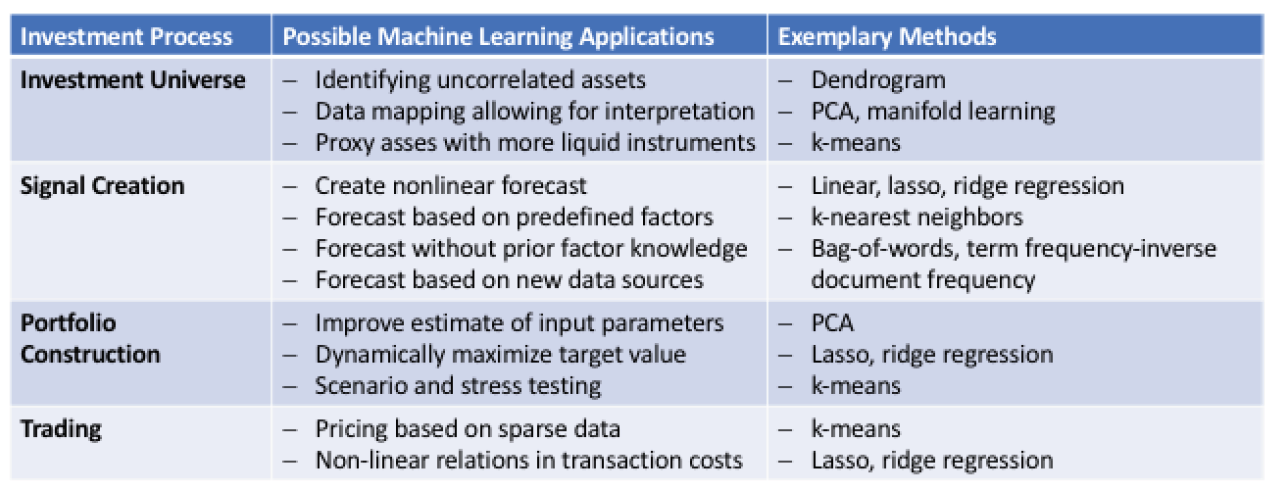
However, the inherently flexible nature of machine learning methods is also the biggest challenge. It requires that the methods are put in the right context and thoughtfully applied so as to solve questions in a way that produces meaningful outcomes. It would be illusory to believe that artificial intelligence can develop a profitable investment rationale on its own. Accordingly, the authors of the note are convinced that machine learning will most likely not turn out to be a holy grail, but that it will help quantitative investment managers in further improving their allocation processes. Nevertheless, the use of artificial intelligence in asset management is undoubtedly still at an early stage.
Authors Urs Schubiger, Egon Ruetsche, and Fabian Dori from Aquila Capital start with a general overview of machine learning, then elaborate on specific applications in quantitative asset management, highlighting the limitations, challenges and possible remedies before reaching conclusions.