In financial news, authors often offer causal statements to explain why market movements are happening: why inflation is surging or declining, why some companies increase profits and valuation, why others face difficult times, etc…
Each of these causal statements represents a piece of knowledge that the author is sharing as an insight into the forces driving the markets. These statements can be original to the author, or just repeated from another author. In all cases, they represent what an author considered a valuable insight supporting his thesis.
The human readers of these news will gather thousands of such statements as knowledge and will aggregate those into their own thesis over time, with volume limitations coming from their available time to read, and their limited access to worldwide news in multiple languages. Once they have a formalized thesis, they may want to perform some sensitivity analysis on this thesis, and the most mathematically oriented users will leverage Bayesian Belief Network modeling tools. This will require that they model the different variables in play, the relationships between these variables, the marginal and conditional probabilities of these variables, before being able to use such a model for decision support.
New research demonstrates the advantages of a fully automated solution resulting from the combined offerings of two companies, Causality Link and Bayesia. This solution leverages Natural Language Processing (NLP) on tens of millions of financial texts to collect such causal statements, performs an automatic analysis to generate on demand dedicated Bayesian Network definitions focused on specific macro-economic or company targets, and transforms these models into editable and executable Bayesian Networks for analysis and simulation purposes.
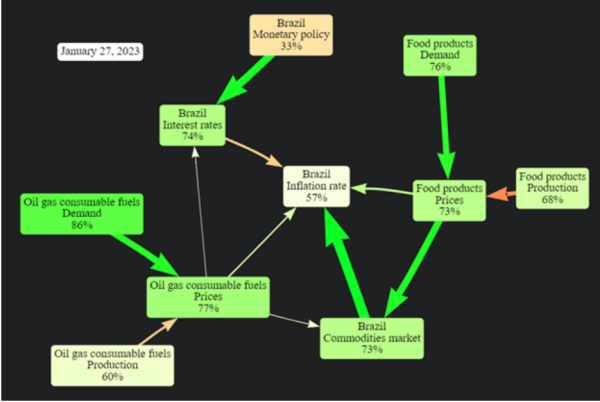
The paper also presents an example of how Bayesian Networks have been used in BayesiaLab to analyze the factors influencing the Brazil inflation rate. The graphical representation of the Bayesian Network makes it easy to interpret the causal relationships between factors such as interest rates and commodity prices. Furthermore, the transparency of the causal links in the model provides further insight into the collective intelligence around the topic.