Although many banks, particularly those operating in jurisdictions with stringent regulatory requirements, have validation frameworks and practices in place to assess and mitigate the risks associated with traditional models, these are often insufficient to deal with the risks associated with machine-learning models.
Conscious of the problem, many banks are proceeding cautiously, restricting the use of machine-learning models to low-risk applications. Their caution is understandable given the potential financial, reputational, and regulatory risks.
Banks could, for example, find themselves in violation of anti-discrimination laws, and incur significant fines: a concern that pushed one bank to ban its HR department from using a machine-learning résumé screener. A better approach, however, and ultimately the only sustainable one if banks are to reap the full benefits of machine-learning models, is to enhance model-risk management.
Regulators have not issued specific instructions on how to do this. In the United States, they have stipulated that banks are responsible for ensuring that risks associated with machine-learning models are appropriately managed, while stating that existing regulatory guidelines, such as the Federal Reserve’s “Guidance on Model Risk Management” (SR11-7), are broad enough to serve as a guide.
Machine learning models typically act on vastly larger data sets, including unstructured data such as natural language, images, and speech. The algorithms are typically far more complex than their statistical counterparts and often require design decisions to be made before the training process begins. And machine learning models are built using new software packages and computing infrastructure that require more specialized skills.
The response to such complexity does not have to be overly complex, however. If properly understood, the risks associated with machine-learning models can be managed within banks’ existing model-validation frameworks, as the exhibit below illustrates.
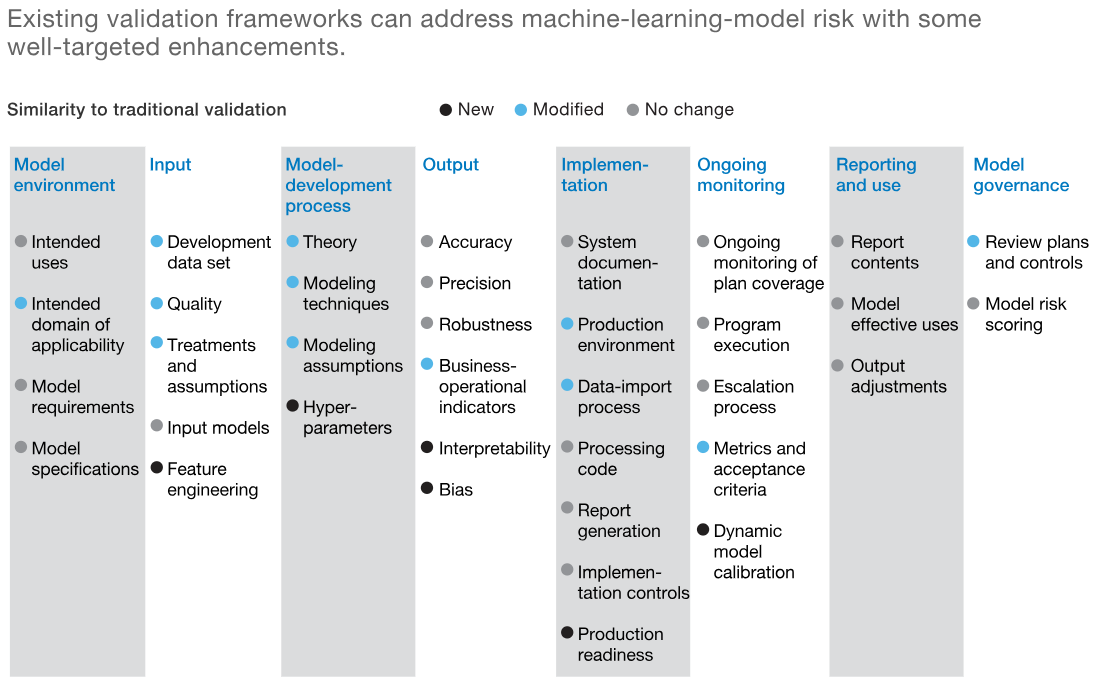
Banks will need to proceed gradually. The first step is to make sure model inventories include all machine learning–based models in use. You may be surprised to learn how many there are. One bank’s model risk-management function was certain the organization was not yet using machine-learning models, until it discovered that its recently established innovation function had been busy developing machine-learning models for fraud and cybersecurity.