Researchers from Cornell, Tsinghua and Beihang universities directly optimize the objectives of portfolio management via reinforcement learning — an alternative to conventional supervised learning-based paradigms that entail first-step estimations of return distributions, pricing kernels, or risk premia.
Building upon breakthroughs in artificial intelligence (AI), they develop multi-sequence neural network models tailored to distinguishing features of economic and financial data, while allowing training without labels and potential market interactions. The resulting AlphaPortfolio yields stellar out-of-sample performances (e.g., Sharpe ratio above two and over 13% risk-adjusted alpha with monthly re-balancing) that are robust under various economic restrictions and market conditions (e.g., exclusion of small stocks and short selling).
They project AlphaPortfolio onto simpler modeling spaces (e.g., using polynomial-feature-sensitivity) to uncover key drivers of investment performance, including their rotation and nonlinearity. More generally, they highlight the utility of deep reinforcement learning in finance and invent “economic distillation” tools for interpreting AI and big data models.
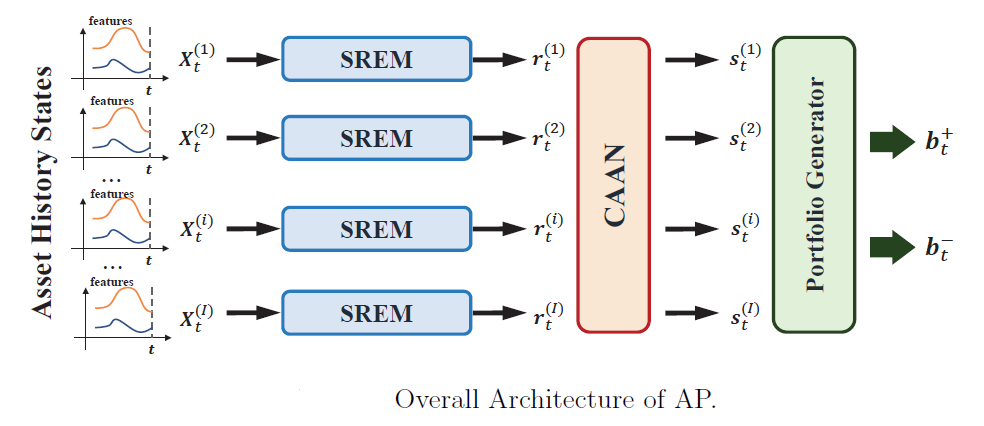